FINN
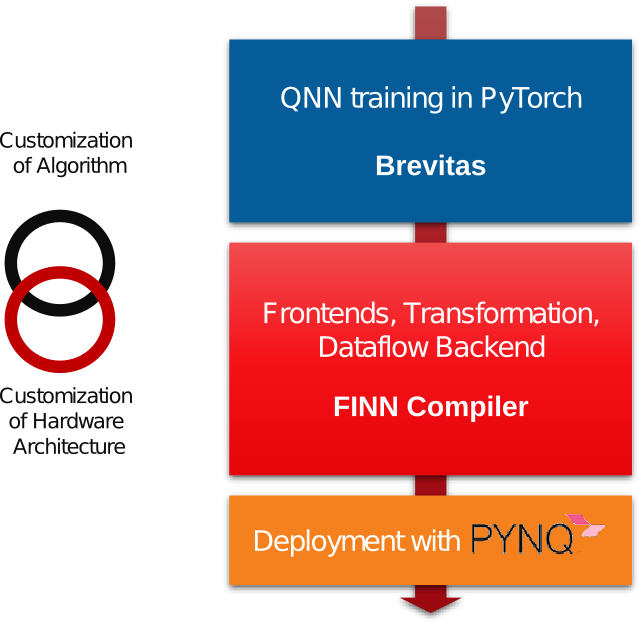
FINN is an
experimental framework from Xilinx Research Labs to explore deep neural network
inference on FPGAs.
It specifically targets quantized neural
networks, with emphasis on
generating dataflow-style architectures customized for each network.
It is not
intended to be a generic DNN accelerator like xDNN, but rather a tool for
exploring the design space of DNN inference accelerators on FPGAs.
A new, more modular version of FINN is currently under development on GitHub, and we welcome contributions from the community!
Quickstart
Depending on what you would like to do, we have different suggestions on where to get started:
- I want to try out prebuilt QNN accelerators on real hardware. Head over to BNN-PYNQ repository to try out some image classification accelerators, or to LSTM-PYNQ to try optical character recognition with LSTMs.
- I want to train new quantized networks for FINN. Check out Brevitas, our PyTorch library for training quantized networks. The Brevitas-to-FINN part of the flow is coming soon!
- I want to understand the computations involved in quantized inference. Check out these Jupyter notebooks on QNN inference. This repo contains simple Numpy/Python layer implementations and a few pretrained QNNs for instructive purposes.
- I want to understand how it all fits together. Check out our publications, particularly the FINN paper at FPGA'17 and the FINN-R paper in ACM TRETS.